Quantum computing is set to revolutionize technology by vastly enhancing artificial intelligence capabilities, transforming industries, and reshaping the future. This article provides a comprehensive overview of Quantum Computing & Artificial Intelligence (Quantum AI), highlighting its potential, current applications, and future trends.
Overview of Quantum Computing
Quantum computing is a revolutionary technology leveraging the principles of quantum mechanics, such as superposition and entanglement, to perform computations far beyond the capabilities of classical computers. Unlike classical computers using binary bits, quantum computers use quantum bits, known as qubits, which can exist simultaneously as both 0 and 1. This unique property exponentially expands the computational space, enabling powerful and complex data processing.
Definition and Concepts
At the heart of quantum computing are key quantum principles such as superposition, where qubits can represent multiple states simultaneously, and entanglement, where qubits become interconnected and instantaneously affect each other regardless of distance. These capabilities underpin quantum algorithms that could vastly outperform classical computing methods in specific applications.
Investment and Market Growth
The quantum computing market is experiencing unprecedented growth, surpassing global investments of $1 billion in 2024. Quantum computing hardware, particularly superconducting qubits, captures around 60% of this investment. Predictions estimate that quantum computing could generate annual value creation between $5 to and $10 billion before 2030 and potentially up to $850 billion post-2040.
Key Takeaways
- Quantum computing uses qubits to perform complex computations beyond classical computers.
- The quantum market is rapidly growing with significant investment and projected value.
- Quantum AI combines quantum computing and AI to enhance efficiency and processing.
- Quantum computing benefits AI through faster processing, optimization, and data analysis.
- Quantum AI applications span NLP, ML, chemistry, finance, healthcare, and security.
- Challenges include hardware limitations, integration issues, scalability, ethics, and regulation.
- The future predicts a quantum advantage in the 2030s and hybrid models dominating.
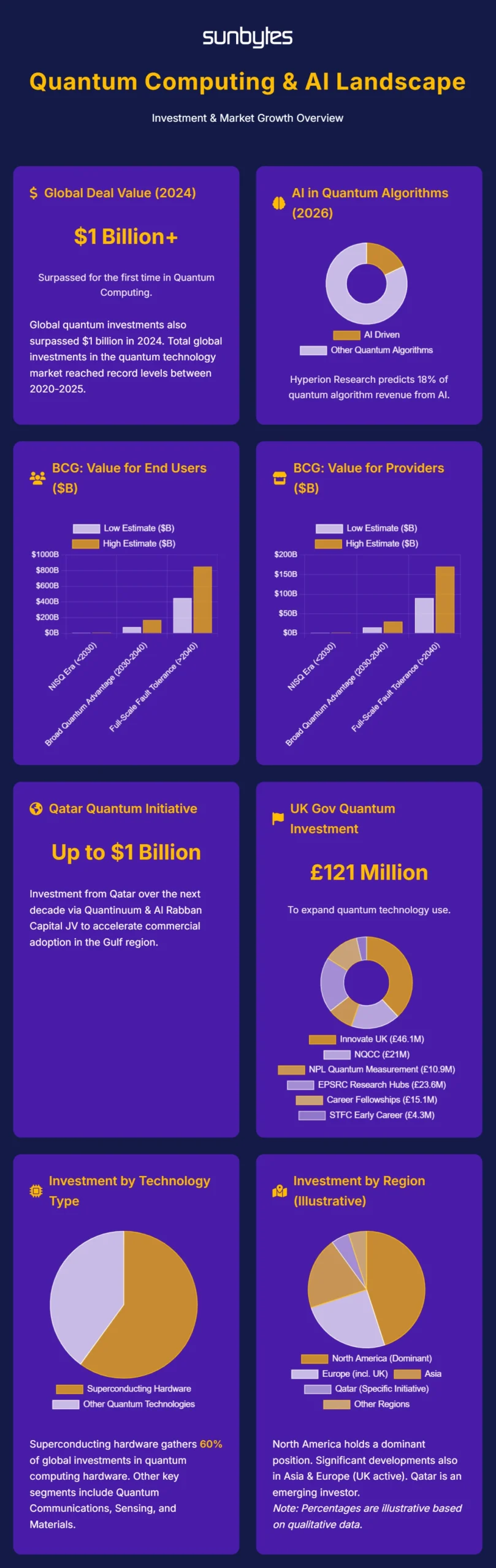
The Process of Conducting Quantum Computing
Quantum computing involves encoding information in qubits, manipulating them using quantum gates, and managing quantum error correction. Given the fragile nature of qubits, quantum error correction and mitigation strategies are crucial. Measurement processes then collapse these quantum states into deterministic results, often complemented by classical post-processing to derive actionable insights.
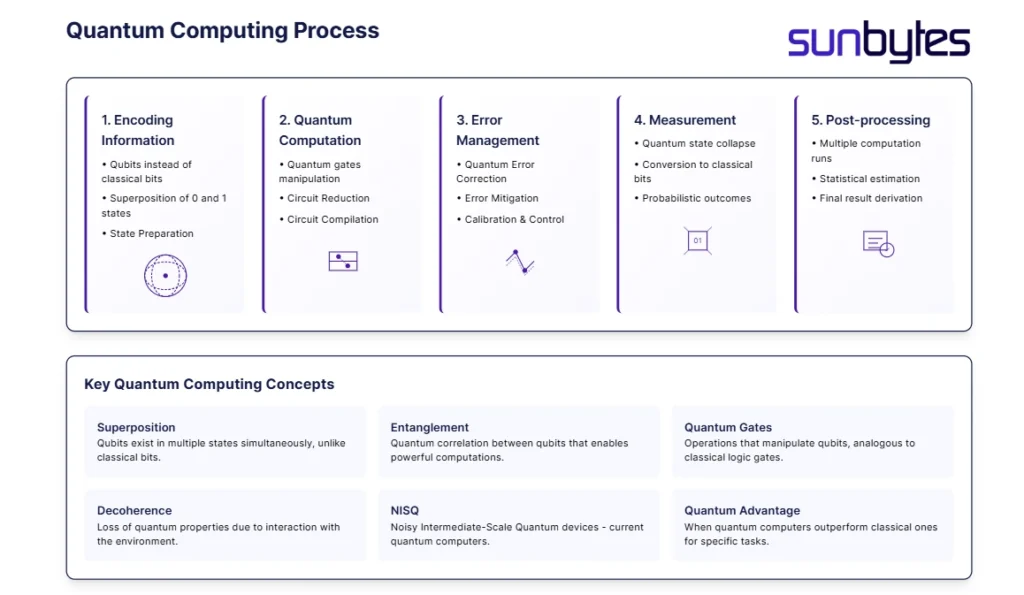
The Intersection of Quantum Computing and Artificial Intelligence (Quantum AI)
It is important to note that the relationship is synergistic, with AI playing an important role in the advancement of quantum computing technology. Classical computing, the foundation of traditional computers, relies on bits representing information as 0 or 1. Artificial intelligence (AI) aims to mimic human intelligence in machines, enabling tasks like learning and problem-solving through data analysis using algorithms. AI is now commonplace, with recent progress in machine learning (ML) and deep learning largely fueled by enhancements in classical computing hardware, notably GPUs, which have facilitated the training of large language models (LLMs). Classical computing provides the necessary processing power for AI algorithms to recognize patterns and make predictions.
However, classical AI methods are facing limitations in computational power for increasingly complex tasks. Today’s supercomputers and cloud servers consume substantial electrical power to manage the extensive computations required for advanced AI.
How Quantum Computing Affects AI Development
Quantum AI is where quantum computing intersects with artificial intelligence (AI), promising breakthroughs in efficiency, scalability, and processing power. Quantum algorithms could significantly enhance AI models, transforming industries from healthcare to finance.
Enhanced Performance and Efficiency
Quantum computers offer a more sustainable, efficient, and high-performance approach to AI, potentially decreasing costs and improving scalability. By utilizing quantum superposition, entanglement, and interference, quantum AI models could significantly reduce both computational cost and energy consumption, with some systems showing dramatic reductions compared to classical supercomputers.
Faster Data Processing and Model Training
Quantum computers can process multiple data points concurrently and perform complex matrix operations at a faster rate than classical systems. This capability promises to drastically reduce the training time for AI models and enable the handling of much larger and more complex datasets.
Improved Optimization
Quantum computing is well-suited for tackling computationally intensive optimization problems. Algorithms like QAOA and VQE could enhance AI optimization tasks, such as fine-tuning machine learning models.
Better Data Analysis and Pattern Recognition
Quantum machine learning algorithms have the potential to uncover intricate patterns in data that classical algorithms may miss. They can process and classify large datasets more efficiently, potentially accelerating machine intelligence techniques.
Overcoming Classical Hardware Limits
Quantum computers may enable AI to address currently intractable problems for classical computers, such as breakthroughs in drug discovery and materials science through accurate simulations of chemical reactions.
Advancements in Natural Language Processing (NLP)
Quantum Natural Language Processing (QNLP) aims to adapt classical NLP techniques like word embeddings, recurrent neural networks, and transformers for quantum computers. This could lead to more efficient processing of language structures and improved AI assistants. Early research suggests that quantum models can achieve results comparable to classical models on language tasks.
Enhanced Security and Privacy for AI
Quantum computing has the potential to improve AI security through advancements in encryption and cybersecurity, including quantum key distribution (QKD) and blind quantum computing.
Challenges and Limitations of Quantum Computing and Artificial Intelligence
The landscape of Quantum computing and artificial intelligence (Quantum AI), while promising, is marked by significant challenges and limitations across various domains:
Technical Limitations of Quantum Hardware
Quantum computing faces substantial hurdles, including qubit fragility leading to decoherence, the considerable challenge and expense of error correction necessitating numerous qubits, and difficulties in qubit engineering and entanglement. Iterative error mitigation adds further cost. Building and maintaining quantum hardware is expensive due to extreme cooling needs and demanding high-precision manufacturing and testing. Current metrics like qubit count are insufficient indicators of performance, as quantum computations are more prone to errors and incur significant control burdens and overhead costs.
Integrating Quantum Computing and AI
Integrating quantum computing and artificial intelligence faces several key difficulties:
- The development of AI algorithms compatible with quantum hardware is complex and necessitates specialized expertise.
- Combining performance features to ensure effective algorithm operation presents a significant challenge.
Limitations of Quantum AI Algorithms and Scalability
Current quantum AI approaches encounter substantial limitations:
- Quantum neural networks suffer from barren plateaus.
- Effectively managing large datasets requires millions of qubits, a capacity far exceeding current quantum hardware.
- Quantum recommendation algorithms face timeliness issues as the number of participants grows.
- Improving the scalability of simulations for intricate systems remains a critical need.
Ethical and Societal Implications
Quantum AI’s transformative potential raises critical ethical concerns. Enhanced surveillance capabilities could infringe privacy and civil liberties, and quantum encryption methods introduce cybersecurity risks, requiring robust post-quantum cryptography solutions. Ensuring equitable access to quantum AI technologies remains a crucial societal challenge, alongside managing job displacement due to automation.
Business and Regulatory Hurdles
Addressing the ethical and societal challenges of Quantum AI necessitates interdisciplinary collaboration. The interpretability of these models is hindered by their “black box” nature, raising concerns about the right to explanation. Replicability is challenging due to the probabilistic nature and noise inherent in quantum computing. The technology’s complexity and rapid evolution make regulation difficult, emphasizing the need for individual ethical frameworks.
Clear principles for responsible use—including transparency, fairness, security, accountability, and bias prevention—are essential. Businesses risk ignoring Quantum AI. The continuous development of this field introduces new complexities that demand ongoing monitoring and strategic evaluation.
Current Applications of Quantum Computing and Artificial Intelligence (Quantum AI)
Quantum AI applications are expanding across sectors.
Natural Language Processing (NLP)
Rebuilding ML techniques using quantum computers, including quantum word embeddings and transformer models like “Quixer,” shows promise for enhanced chatbots and translators by leveraging the rich representation of complex numbers for language’s structure.
Machine Learning (ML)
Research includes quantum neural networks, support vector machines, and principal component analysis, aiming for more efficient processing of large datasets. Variational Quantum Algorithms (VQE) have demonstrated good performance on NISQ devices for Quantum Artificial Intelligence (QAI) tasks.
Optimization
Quantum computing’s ability to explore numerous possibilities simultaneously can improve AI optimization tasks, such as route selection for delivery trucks, potentially through algorithms like the Quantum Approximate Optimization Algorithm (QAOA).
Chemistry and Materials Science
Quantum computing and artificial intelligence are expected to accelerate drug discovery by improving molecule identification and chemical reaction simulations, leading to new treatments. It can also enhance pharmaceutical research by providing better training data for ML models and aid in predicting properties of new chemicals and discovering new materials.
Finance
Applications include reshaping risk assessment, portfolio optimization, improving fraud detection, and potentially predicting market trends with greater accuracy.
Healthcare
Beyond drug discovery, Quantum computing and artificial intelligence could advance personalized medicine by analyzing complex biological systems for tailored treatments.
Robotics
Quantum AI may accelerate computations, improve sensing capabilities, enhance image processing, and optimize robotic machine motion through quantum-reinforced learning.
Data Security
Advancements in encryption and cybersecurity through quantum computing can enhance AI security, although the future ability of quantum computers to break traditional encryption poses a challenge.
IQM Quantum Computers is specifically focused on developing quantum processors and exploring ML use cases, including AI for auto-calibration, error correction/mitigation, algorithm optimization, and chip design, while Quantinuum emphasizes Generative Quantum AI and rebuilding ML techniques for NLP with their full-stack approach. Cloud-based quantum computing platforms from IBM, Google, Microsoft, and AWS also support hybrid quantum-classical AI research. QAI Ventures invests in Quantum and AI initiatives.
Future Trends in Quantum Computing and Artificial Intelligence (Quantum AI)
BCG analysis predicts a broad quantum advantage in the early 2030s and full-scale fault tolerance after 2040. Quantum AI breakthroughs are anticipated by the late 2020s or early 2030s, coinciding with the expected availability of error-corrected quantum computers with tens to hundreds of logical qubits. The full implementation of quantum computing in areas such as automation, energy, computer security, and complex mathematical chemistry and drug design may take over a decade.
Hybrid quantum-classical computing models will likely dominate, combining classical computing efficiency with quantum computing’s advanced processing capabilities. Quantum transformers, a quantum version of classical transformer models, may significantly enhance AI capabilities, reducing energy consumption and computational load.
Quantum computing and artificial intelligence together herald an unprecedented technological revolution. Although Quantum AI faces limitations, its potential to transform computing, processing capabilities, and industry-specific applications is undeniable. As quantum research advances and quantum computing ecosystems mature, Quantum AI stands poised to redefine the future landscape of technology and artificial intelligence.
Frequently Asked Questions
Quantum computing is a revolutionary technology that uses quantum mechanics principles like superposition and entanglement to perform complex computations beyond the capabilities of classical computers. It uses qubits, which can exist as both 0 and 1 simultaneously, instead of bits.
Classical computers use bits that represent either 0 or 1. Quantum computers use qubits, which can represent both 0 and 1 at the same time due to superposition, allowing for much more complex computations.
Qubits, or quantum bits, are the basic units of information in quantum computing. Unlike classical bits that are either 0 or 1, qubits can be 0, 1, or both simultaneously due to superposition.
Quantum AI refers to the intersection of quantum computing and artificial intelligence. It aims to use quantum computers to enhance AI models, improve efficiency, scalability, and processing power.
Quantum computing can enhance AI through faster data processing, improved optimization, better data analysis and pattern recognition, and by overcoming limitations of classical hardware. It can also enhance Natural Language Processing (NLP) and improve security and privacy for AI.